PointNet: 基於3D點雲之深度學習網路用於分類及語意分割
PointNet: 基於3D點雲之深度學習網路用於分類及語意分割 [1]
呂紹篁 同學
呂紹篁 同學
隨著2D影像語意分割(Semantic Segmentation)之研究漸趨飽和,以及3D感測器愈來愈容易取得,然而在進行點雲(Point Cloud)之分類時,往往只能使用較手工的特徵如FPFH(Fast PointFeature Histograms)[2] 及SIFT(Scale-Feature Transform) [3],或者像3D CNN [4] 將點雲數據轉成體素(Voxelgrid)再進行卷積,沒有一個深度網路能夠直接處理無序的點雲資料。 史丹佛大學的Charles等人提出一個能夠輸入無序之點雲資料,並輸出點雲之語意分割之深度網路,網路架構如下圖。
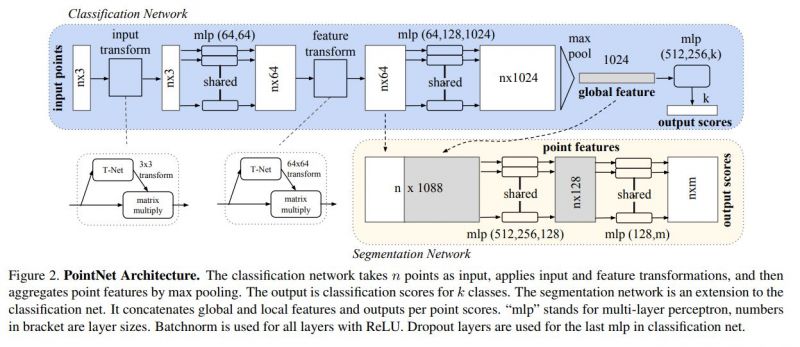
圖一、深度網路架構
作者以同樣使用點雲輸入,透過傳統特徵萃取進行MLP的演算法作為比較對象,做成表一及表二,可以發現在分類工作上,類別平均準確度上有高達10%的進步,而語意分割部份則幾近兩倍的進步。
表一、ModelNet40 分類結果
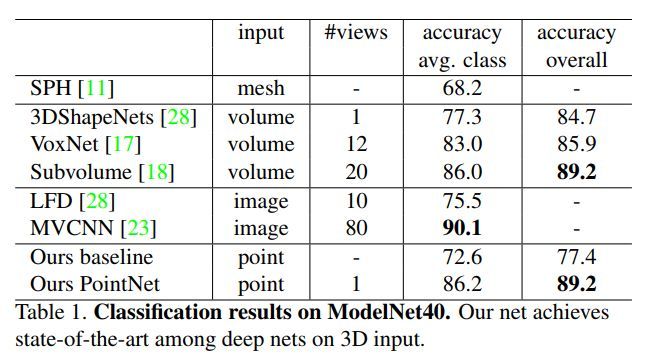
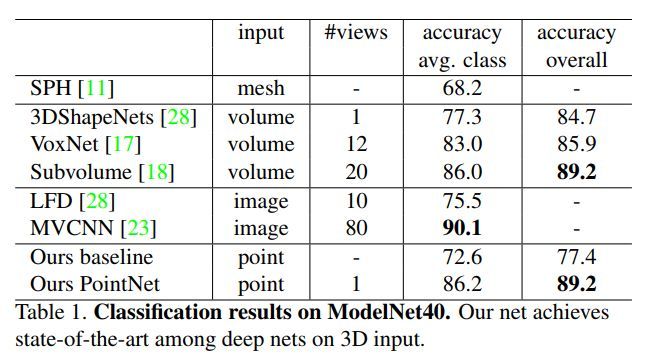
表二、語意分割結果
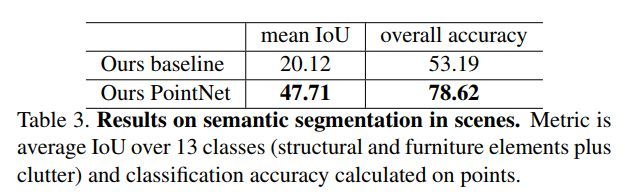
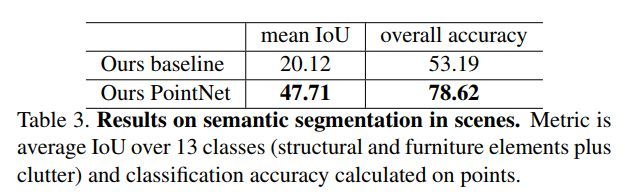
且該網路具有強大的穩健性,在圖二中作者針對點雲進行資料量的改變(增加/減少),可以發現在下圖中,就算資料量已減少一半,其分類準確性還是有將近90%的水準。
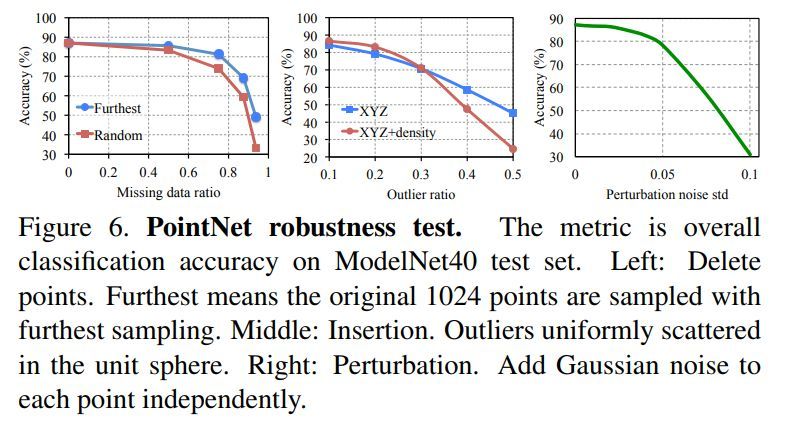
圖二、PointNet 穩健性測試
總結以上,該神經網路能夠準確分類點雲資料,且網路上也有基於Caffe及Tensor Flow之開放原始碼甚至Docker映像檔提供給需要的研究人員使用。
參考文獻:
[1] Qi, Charles R., et al. "Pointnet: Deep learning on point sets for 3d classification andsegmentation." Proc. Computer Vision and Pattern Recognition (CVPR), IEEE 1.2 (2017): 4.
[2] Rusu, Radu Bogdan, Nico Blodow, and Michael Beetz. "Fast point feature histograms(FPFH) for 3D registration." Robotics and Automation, 2009. ICRA'09. IEEE InternationalConference on . IEEE, 2009.
[3] Lowe, David G. "Object recognition from local scale-invariant features." Computer vision,1999. The proceedings of the seventh IEEE international conference on . Vol. 2. Ieee,1999.
[4] Qi, Charles R., et al. "Volumetric and multi-view cnns for object classification on 3d data."Proceedings of the IEEE conference on computer vision and pattern recognition . 2016.
參考文獻:
[1] Qi, Charles R., et al. "Pointnet: Deep learning on point sets for 3d classification andsegmentation." Proc. Computer Vision and Pattern Recognition (CVPR), IEEE 1.2 (2017): 4.
[2] Rusu, Radu Bogdan, Nico Blodow, and Michael Beetz. "Fast point feature histograms(FPFH) for 3D registration." Robotics and Automation, 2009. ICRA'09. IEEE InternationalConference on . IEEE, 2009.
[3] Lowe, David G. "Object recognition from local scale-invariant features." Computer vision,1999. The proceedings of the seventh IEEE international conference on . Vol. 2. Ieee,1999.
[4] Qi, Charles R., et al. "Volumetric and multi-view cnns for object classification on 3d data."Proceedings of the IEEE conference on computer vision and pattern recognition . 2016.